
In the context of building acoustics and the acoustic diagnosis of an existing room, this paper introduces and investigates a new approach to estimate mean absorption coefficients solely from a room impulse response (RIR). Comparisons of the proposed approach with severalĮxisting methods reveal that the combination of acoustic-space learning withīayesian inference enables our method to outperform state-of-the-art methods. (VESSL) yields a Bayesian estimation of the 2D locations and time-frequency The associatedĪlgorithm, referred to as variational EM for source separation and localization Sound sources and we propose a variational EM framework. We further generalize the model to the challenging case of multiple Spectrograms, and hence for 2D localization of natural sound sources such as WeĮxtend this solution to deal with missing data and redundancy in real world
#Soundsource macon ga full#
Obtaining the full posterior density function of a sound source direction. Procedure for estimating the model parameters, followed by Bayes inversion for We derive a closed-form expectation-maximization (EM) Propose a probabilistic piecewise affine mapping model (PPAM) specificallyĭesigned to deal with high-dimensional data exhibiting an intrinsic piecewise Motor states of the listener, or equivalently, the sound source directions.
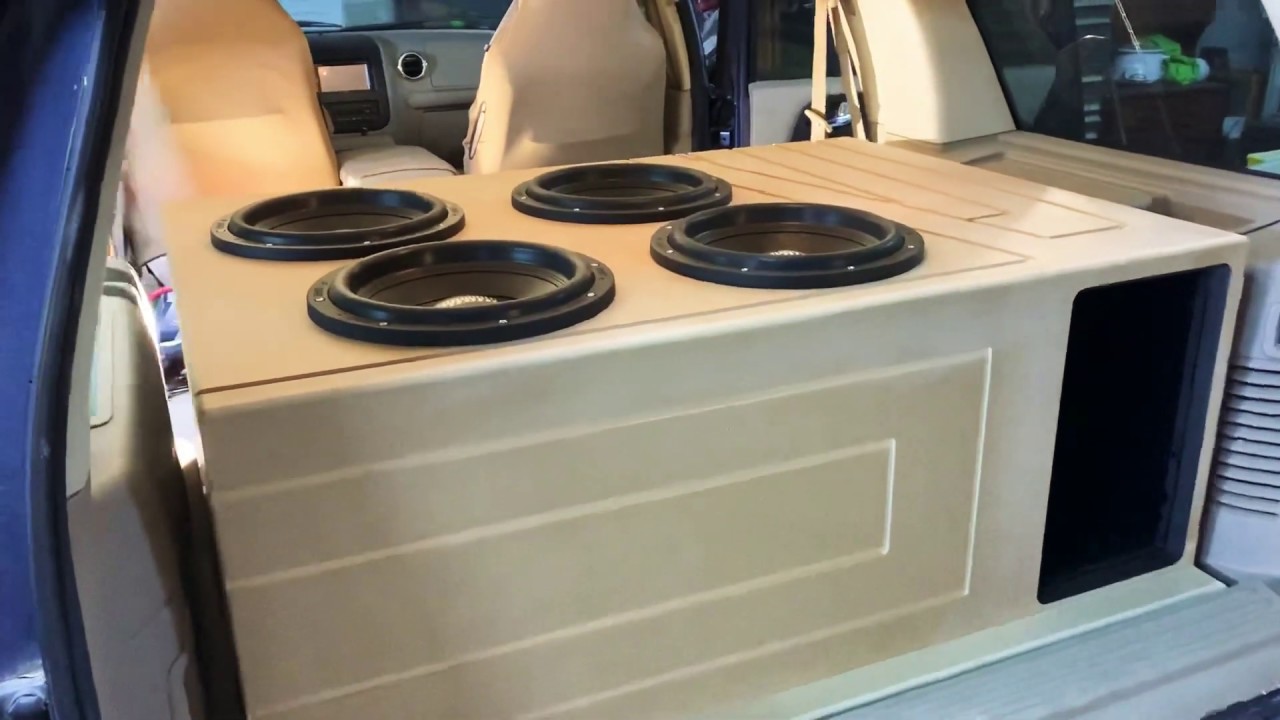
These data lie on a two-dimensional (2D) smooth manifold parameterized by the

A non-linear dimensionality reduction technique is used to show that Spectral data, based on a corpus recorded with a human-like audiomotor robot The latent low-dimensional structure of the high-dimensional interaural To introduce the binaural manifold paradigm.

We lay theoretical and methodological grounds in order The localization and separation of multiple sources that simultaneously emit Generated by a full-spectrum sound source and of using the learned model for In this paper we address the problems of modeling the acoustic space
